There's a paradox inherent to being a data leader in 2025: you have to simultaneously be an optimist who believes in miracles and a realist dealing in cold, hard facts.
The miracle is that it’s a transformative time in ML & AI: they’re increasingly capable of game-changing outcomes, and the landscape is changing so quickly you need to have contingency plans for possibilities that were previously totally unreachable. It's hard not to find this energizing.
But, the hard fact remains: major data initiatives are risky and take months, if not years, to move from conception to production. Your role is to deliver, not just to write checks that you can’t cash. You're probably not the kind of person who makes wild assertions — yet all of a sudden, it's an expected piece of your role.
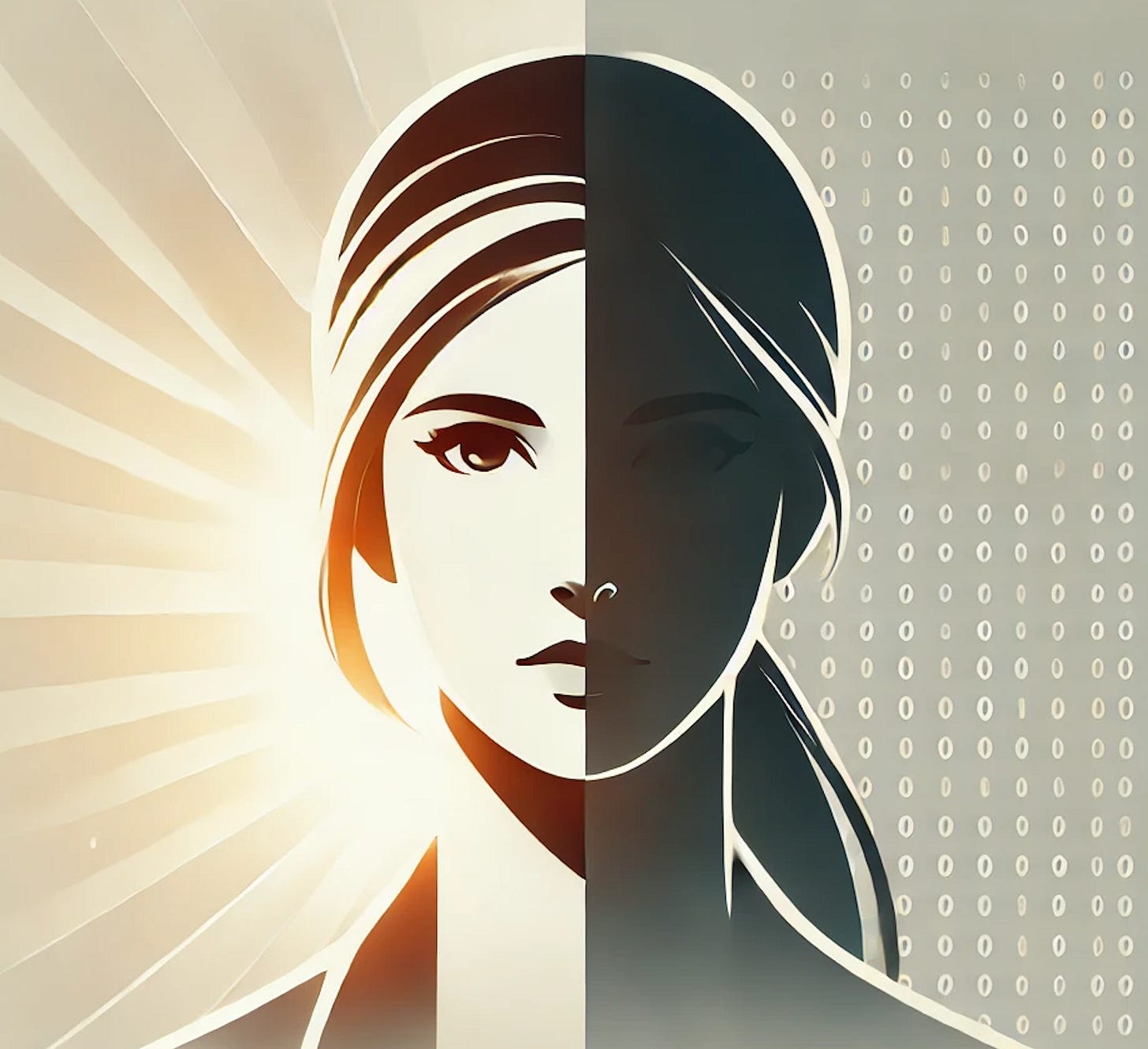
This leaves data leaders in an awkward position as the generative AI hype cycle barrels forward. CEOs and boards are clamoring for big AI wins, while the fundamentals haven’t changed: good work takes time, careful planning, and a steady hand on the wheel. As a data leader, you have to manage both sides of this coin carefully.
When I was at Uber, I was responsible for coming up with targets for my teams like “increase gross bookings volumes by 5%”. These goals always feel a bit like they are coming out of thin air – and they need to jointly satisfy the big dreamers who expect an ML-fueled transformation while actually being achievable. Not ambitious enough? You’ll be out of a job. So ambitious you don’t come close 6 months later? You’ll also be out of a job.
The growing temperature gap
A key challenge here is the growing gap in expectations between the penthouse and the ground floor.
Given the state of energy in AI, things are running hot red right now in the C-suite. Leadership teams read about the latest model’s latest breakthrough or a competitor's bold AI move, and they see transformative possibilities everywhere. The future feels electric with potential — and FOMO.

But the data scientists and engineers — the folks who actually have their hands on keyboard (and in the data) — are running cooler. They're wrestling with the daily painstaking realities of messy data, complex edge cases, and the challenge of getting simple models to scale reliably in production. And unlike traditional tech projects, where progress comes in clear increments, data initiatives can stay in an uncertain state for months as the team iterates on various ideas.
Leaders are left trying to keep both groups aligned. The reality is you need to embrace the delta, because it’s hard to imagine the dramatic expectations gap narrowing anytime soon.
Our advice: build a time-horizon portfolio
The solution isn't to dampen C-suite enthusiasm or forego unproven approaches to data projects. Instead, you need to build and disseminate a strategy which explicitly ladders together a portfolio of bets that span risks and time frames.
Here’s a quick test. In under a minute, can you rattle off a categorized list of:
- Quick wins (<3 months): These are your credibility builders. Think dashboard improvements, model performance tweaks, or targeted data quality fixes. They won't change the world, but they maintain momentum and buy you time for bigger plays.
- Strategic projects (3-12 months): This is where the real value creation happens — new model deployments, data platform upgrades, internal data products, or cross-functional initiatives that solve meaningful business problems. These projects need room to breathe, but they're concrete enough (and easy enough to understand) to keep stakeholders engaged. Derisk these projects as fast as possible, and make sure to use learnings from one project to inform others.
- Transformational bets (12+ months): These align with the C-suite's visionary goals. Think enterprise-wide AI infrastructure or novel applications that could reshape your business. The key is to keep these connected to reality — they should build naturally from your strategic projects, not float disconnected in dream-space.
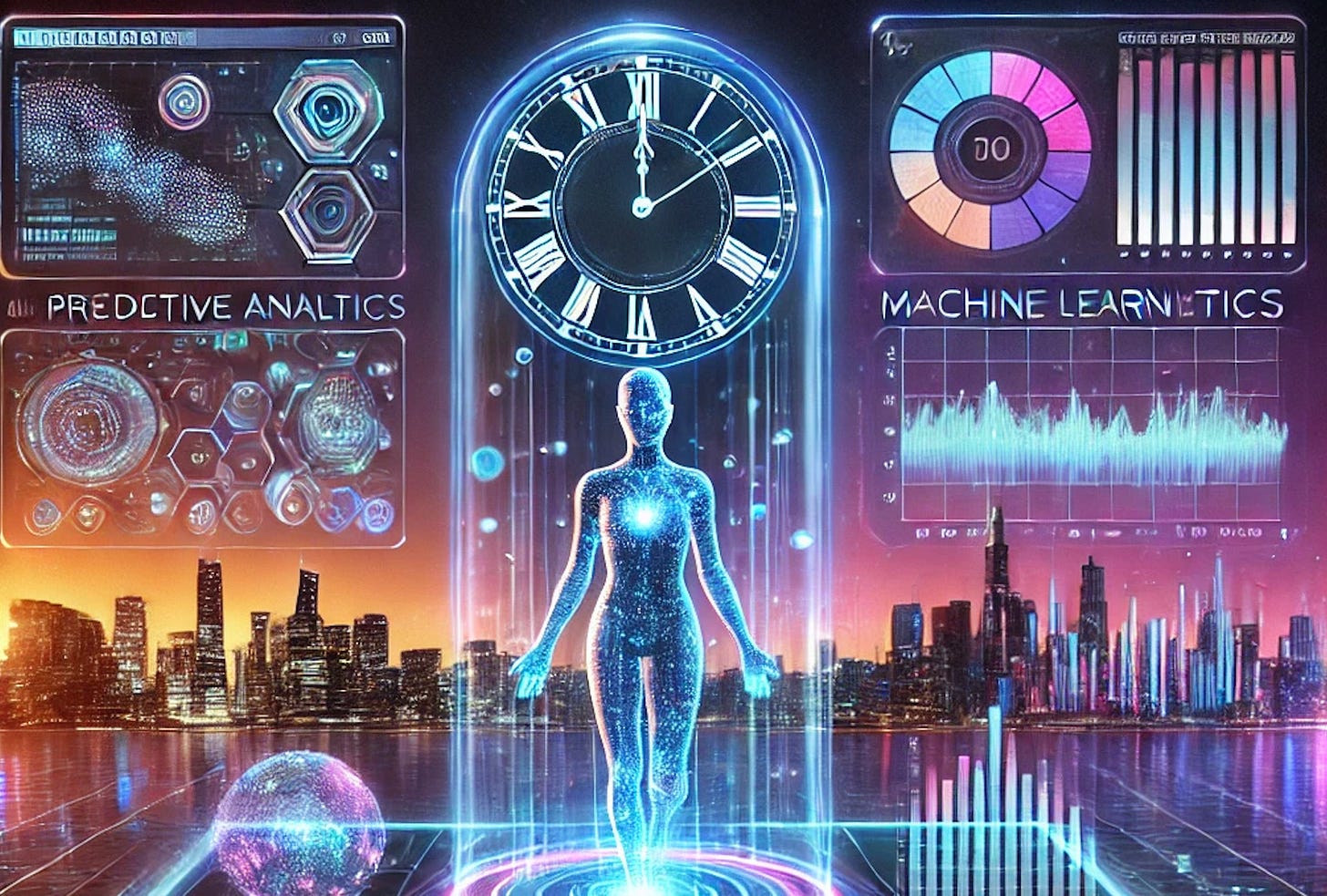
For example, my list at Uber might have included:
- Upgrading area-level metrics and incrementality tradeoffs to reflect the latest priorities (quick, high visibility win)
- Moving ML models from batch to real-time processing (strategic)
- Marrying realtime pricing and dispatch — two independent systems — together (transformational)
In some ways this is obvious management advice, but my experience from talking to leaders is that they only have their list down cold during planning season; now, as we roll into 2025, many have already lost track.
The magic happens when the layers work together. Your quick wins buy credibility for strategic projects. Your strategic projects lay groundwork for transformational bets. And critically, you can point to progress at every review.
Threading the needle
Success as a data leader means actively managing these different temperatures. That fire in the c-suite can be a great way to get your team resources and visibility. It can be a huge career accelerator for you. But you need to be thoughtful and explicit about your portfolio strategy, and communicate it relentlessly.