
I may be biased, but High Signal hits differently.
Since we launched in October 2024, we’ve hosted conversations with data and AI leaders from industry and academia — including practitioners, advisors, professors, and even several guests who are all three. We’ve been on a mission to bridge the gap between deep-but-theoretical academic perspectives and the blogosphere of content about AIs and LLMs that’s super applied, but not very deep.
If you’re looking to spend your holiday break catching up on the latest in data and AI, start by hearing what the greatest minds in the field have to say — such as:
- Mike Jordan (Berkeley) describing how LLMs are currently terrible at expressing uncertainty, which means the systems we’re building with AI could create a lot of real-world harm
- Gabriel Weintraub (Stanford) explaining how failed experiments teach organizations something very valuable
- Hilary Mason (cofounder, Hidden Door) arguing that while GenAI can automate basic data tasks, human judgment will be essential to frame problems well in the first place
- Chris Wiggins’ (NYT Chief Data Scientist) belief that making predictions alone with ML doesn’t deliver enough value
These are just a few of the highlights from the first seven episodes of the High Signal podcast.
Hosted by our friend Hugo Bowne-Anderson, these are thoughtful interviews with brilliant people, sharing their firsthand experiences and real-world anecdotes — yet always grounded in the deepest understanding of how data and ML actually works. Even though every episode covers a really broad range of topics depending on the guest’s background, you can still pick up some common threads that weave throughout these diverse conversations.
One point of consensus? We’re only in the early days of the data science journey, and teams have enormous opportunities — and challenges — ahead. So read on to check out a highlight from each conversation so far, and dive deeper with the full episodes.
1/ Mike Jordan on AI systems failing at uncertainty
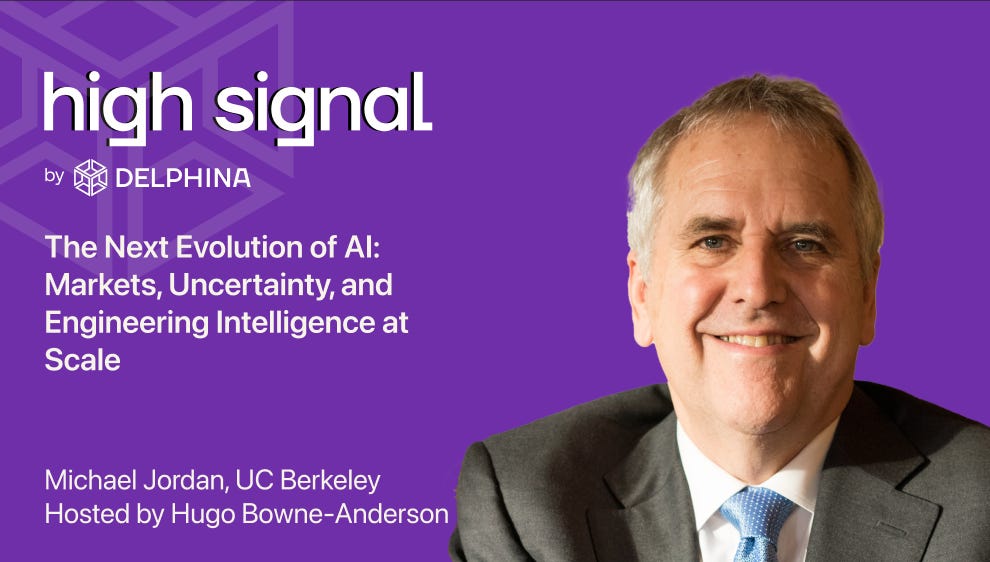
What better guest to launch a data science podcast than the Michael Jordan of ML? Mike is a professor of statistics at UC Berkeley, has a PhD in Cognitive Science, and shared his expansive theories with High Signal on how AI may be evolving to address planetary-level challenges.
He also highlighted one of AI’s biggest challenges: the systems we’re building with GenAI are terrible at expressing uncertainty, which makes them really bad at helping with decision-making. (Kind of a big deal.) Mike illustrates this point about uncertainty using an example from animal behavior: when ducks feed at a pond, they don't simply choose the location with the most food. Instead, they randomize their choices, spreading themselves out in proportion to the food available. While this might seem suboptimal for any individual duck, it creates a better outcome for the group by managing resource scarcity.
This natural behavior, Mike argues, reveals how current AI systems fall short — they're often built to optimize individual decisions without considering systemic effects or resource constraints.
“Uncertainty quantification is a very rich topic, and if you don’t do it, you’re going to build systems that really mess up and hurt people. Some of the dialogue, unfortunately, goes to the idea that ‘There’s never going to be scarcity again because AI is going to create so much wealth.’ Now we’re in the world of science fiction. Sadly, a lot of the so-called thought leaders in the AI world go there very fast — because they don’t really know how to think about this middle work of building and engineering.”
Listen to Mike’s full episode on Apple Podcasts, Spotify, Youtube, or check out the show notes to learn more.
2/ Andrew Gelman on playing vs. writing SimCity
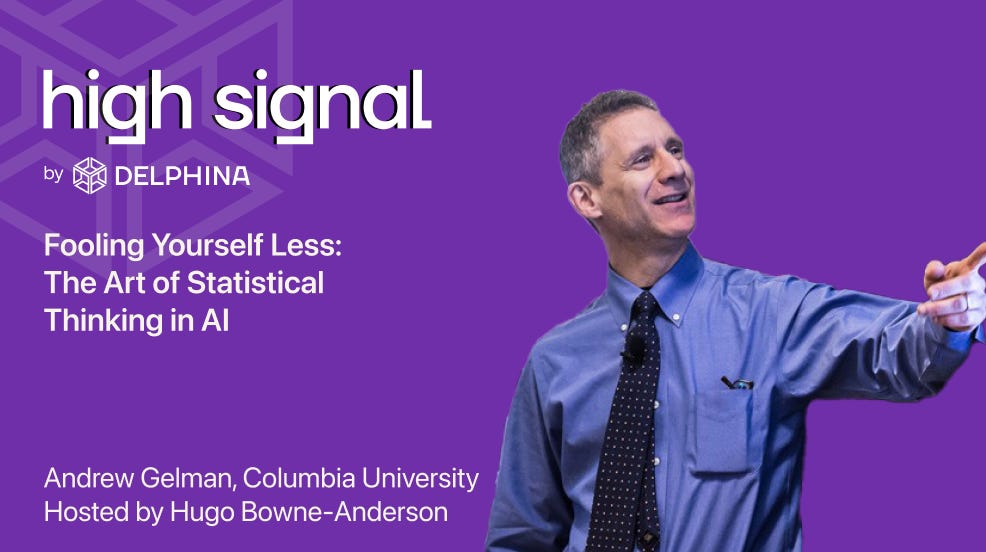
Andrew Gelman, professor of statistics and political science at Columbia University, discussed his perspective on the importance of high-quality data and the critical role casual inference plays in decision-making.
Andrew shared a key part of his own workflow: simulation before data collection. For example, when designing an education experiment to test a new teaching method, Gelman says he would start with simulation — considering realistic effect sizes, accounting for students who won't be affected (those learning primarily through textbooks rather than class instruction), and thinking through real-world factors. This process often reveals sobering insights: expected improvements might be just one or two percentage points, rather than the dramatic changes people hope for.
"I have a rule now: I don't like to ever gather data until I've simulated data first. To analyze data is like playing SimCity, and to simulate data like writing SimCity. It's more work, but it's worth it every time. It's the kind of work that makes you think harder about the problem."
Hear more in Andrew’s full episode on Apple Podcasts, Spotify, Youtube, or check out the show notes.
3/ Chiara Farronato on teaching managers to ask the right questions

Chiara Farronato, associate professor at Harvard Business School, is an expert in marketplace growth strategies. Her research into companies like Uber, Facebook, and Airbnb often includes a critical — and familiar — challenge: helping technical and non-technical teams work together effectively.
She described how her HBS class ‘Data Science for Managers’ attempts to address this very common problem:
“We're not teaching managers to become data scientists — that's for somebody else to do. The final objective is not to teach them statistics but to teach them ways in which they can work effectively with data scientists and engineers. What that means in practice for managers is to ask the right questions, and importantly, understand the answers that they get from their partners."
This is a must listen if you’re a leader (especially a non-DS leader) working closely with data scientists. Hear more in Chiara’s full episode on Apple Podcasts, Spotify, Youtube, or check out the show notes.
4/ Ramesh Johari on why your organization should behave like a toddler

Ramesh Johari has advised tech companies and marketplaces like Uber, Airbnb, and Bumble on developing experimentation platforms — and also happens to be a Stanford professor with degrees in mathematics and computer science from Harvard, Cambridge, and MIT. He brought a unique blend of business acumen and deep technical understanding to our conversation about how companies can evolve from basic experimentation practices to becoming adaptive, self-learning organizations.
He outlined his belief that in order to create a truly data-driven culture, organizations need to push themselves to learn:
"Toddlers are self-learning beings. They experiment in the world around them, learn from it, adapt, evolve, and eventually (hopefully) become high-functioning adults. And I think orgs do the same thing. They take baby steps with experimentation, but in the end, what you're heading towards is a world in which experimentation is augmenting your learning. And I think that self-learning is a North Star to aim for."
Hear more in Ramesh’s full episode on Apple Podcasts, Spotify, Youtube, or check out the show notes.
5/ Gabriel Weintraub on why your experiments should fail
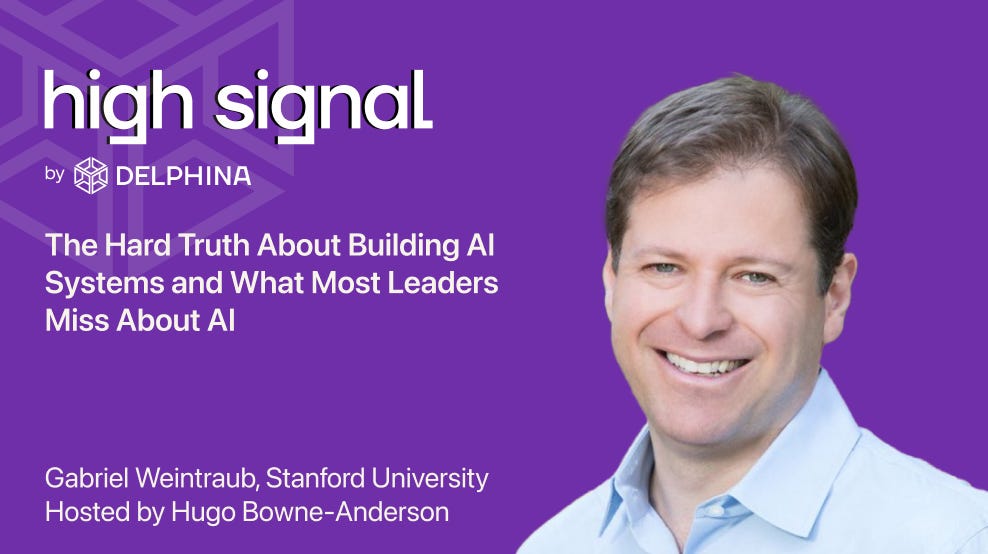
Gabriel Weintraub is the Amman Mineral Professor of Operations, Information, & Technology at Stanford Graduate School of Business — and an advisor to tech companies like Airbnb and AppNexus. He shared refreshingly practical insights with High Signal about the strategies organizations need to have in place before they can build data-driven cultures and use AI in effective ways.
Specifically, Gabriel explained why companies need to conduct experiments that fail:
"Roughly 80 percent of experiments run in tech companies are either flat or negative. A lot of the things we try are not great…but running an experiment that has a flat or negative result is actually helpful because you are learning that what you thought was a good idea is actually not a good idea.”
Get more reassurance about your testing results in Gabriel’s full episode on Apple Podcasts, Spotify, Youtube, or check out the show notes.
6/ Hilary Mason on how to be irreplaceable as a data scientist

Hilary Mason is a data scientist and entrepreneur who left academia to work in startups like Bitly before founding companies like Fast Forward (later acquired by Cloudera) and Hidden Door, which blends generative AI, gaming, and storytelling. As an experienced data leader, she takes a long view on the evolution of data science — and how it will change in the face of GenAI.
“Data science hasn’t ended, but it has changed,” says Hilary. “When things are now branded data science, you don’t get a line out the door for free. A little bit of the ‘shiny’ has gone away. And I think that’s actually really good.”
She goes on to describe how the ability of GenAI to automate many junior-level tasks is forcing the field of data science — among others — to evolve.
“Not just data scientists, but software engineers, or any folks who are in a role where their job would be taking a well-formed problem statement and then doing a repeatable process to answer that question. Any job like that is vulnerable to change. And so you think about, what part is not vulnerable to change? It's good judgement, and the ability to write the problem statement in the first place.”
Learn more from Hilary’s full episode on Apple Podcasts, Spotify, Youtube, or check out the show notes.
7/ Chris Wiggins on aiming higher than prediction

Chris Wiggins is an associate professor of applied mathematics at Columbia University and the Chief Data Scientist at The New York Times. He discussed scaling data functions, the challenges of building robust data systems, and what causes sophisticated AI tools to fall short.
Chris also challenged the status quo around predictions as the outcome of machine learning — saying that data science has to evolve to prescribe meaningful interventions that drive real-world impact.
“In a company, it helps you sleep at night if you can predict accurately which customers are going to cancel their subscription. But ultimately, you’d like to know: What lever do I pull? What is the treatment I should deliver in order to optimize the outcome, rather than merely predicting the outcome in the absence of treatment? …That’s true for figuring out what’s the right marketing message and figuring out what’s the product intervention. Somehow there’s a prescriptive problem that you’d like to get to. And that touches on causal inference in the statistical literature, or it touches on reinforcement learning the machine learning literature…and those two communities are talking to each other more and more.”
Hear Chris’s full episode on Apple Podcasts, Spotify, Youtube, or check out the show notes.
What’s ahead in 2025
Once you’re caught up, stay tuned — we’re starting off the new year with a bang. Our next guests include:
- Elena Grewal, Yale lecturer and an early data science leader at Airbnb
- Guido Imbens, Stanford professor and Nobel Laureate
- Eric Colson, formerly Chief of Algorithms Officer at StitchFix and VP of Data Science and Engineering at Netflix
Subscribe to High Signal on Apple Podcasts, Spotify, and YouTube, or join the conversation on LinkedIn. And don’t hesitate to let us know what guests you’d love to hear from next!